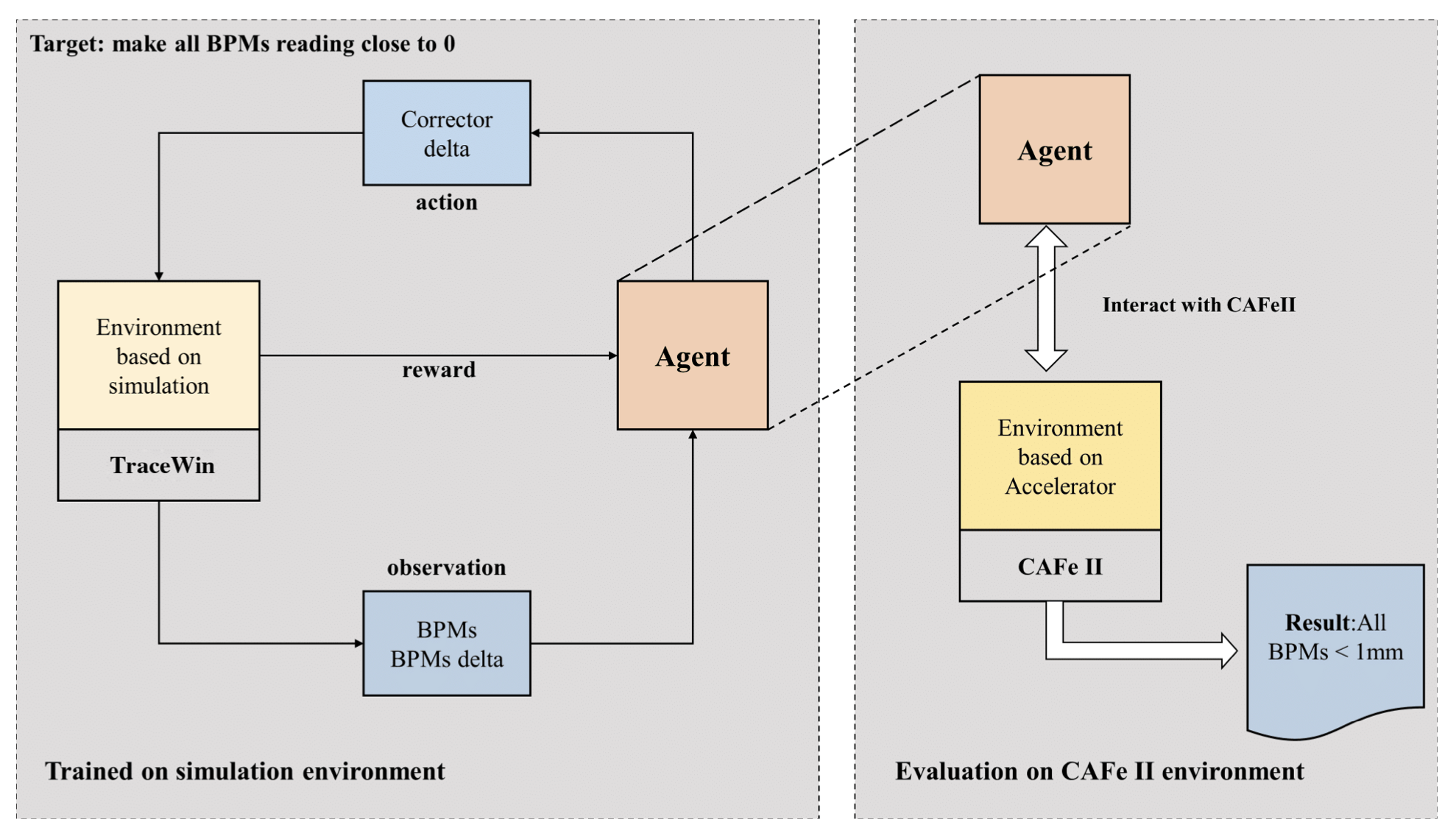
Orbit Correction Based on Improved Reinforcement Learning Algorithm
X. Chen, Y. Jia, X. Qi, Z. Wang, Y. He Chinese Academy of Sciences Physical Review Accelerators and Beams Abstract Recently, reinforcement learning (RL) algorithms have been applied to a wide range of control problems in accelerator commissioning. In order to achieve efficient and fast control, these algorithms need to be highly efficient, so as to minimize the online training time. In this paper, we incorporated the beam position monitor trend into the observation space of the twin delayed deep deterministic policy gradient (TD3) algorithm and trained two different structure agents, one based on physical prior knowledge and the other using the original TD3 network architecture....