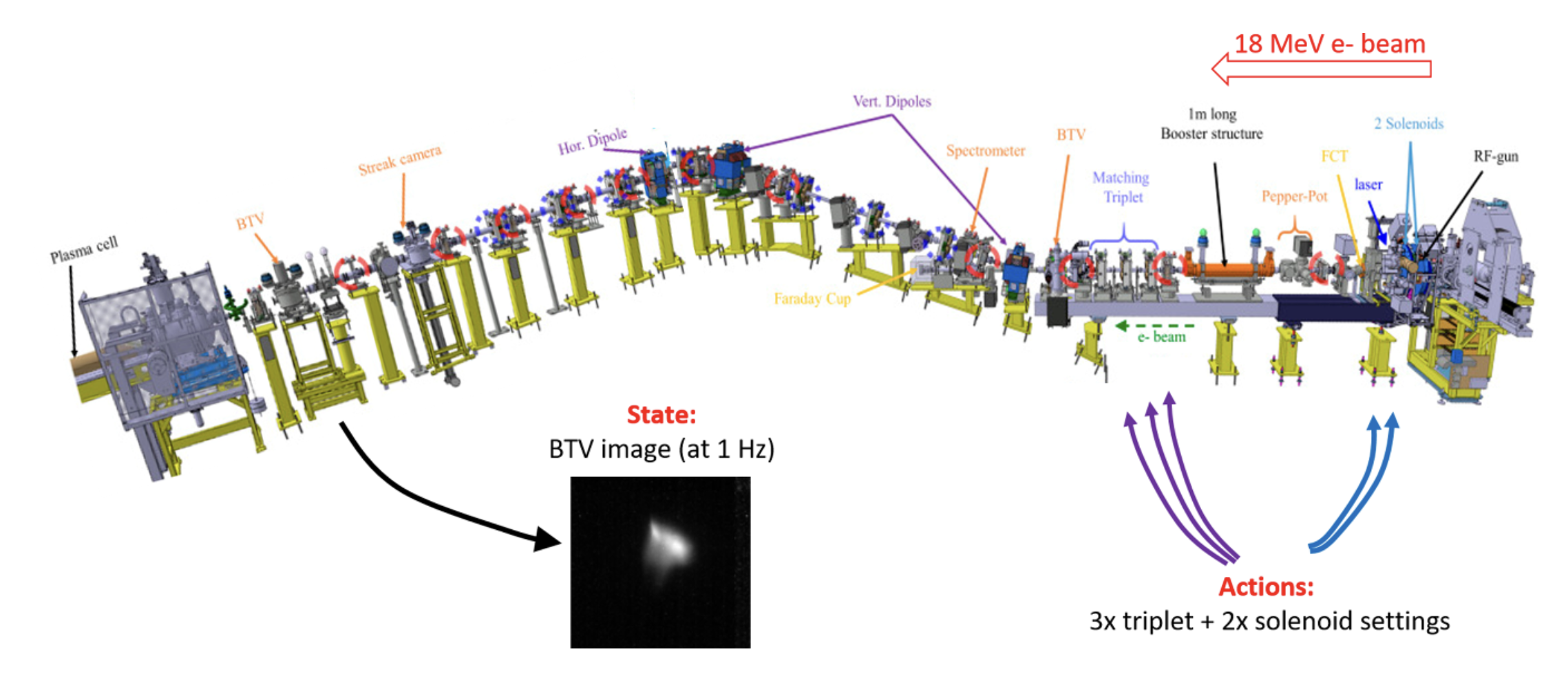
Towards automatic setup of 18 MeV electron beamline using machine learning
F. M. Velotti1, B. Goddard1, V. Kain1, R. Ramjiawan1, G. Z. Della Porta1 and S. Hirlaender2 1CERN, 2University of Salzburg Machine Learning: Science and Technology Abstract To improve the performance-critical stability and brightness of the electron bunch at injection into the proton-driven plasma wakefield at the AWAKE CERN experiment, automation approaches based on unsupervised machine learning (ML) were developed and deployed. Numerical optimisers were tested together with different model-free reinforcement learning (RL) agents....