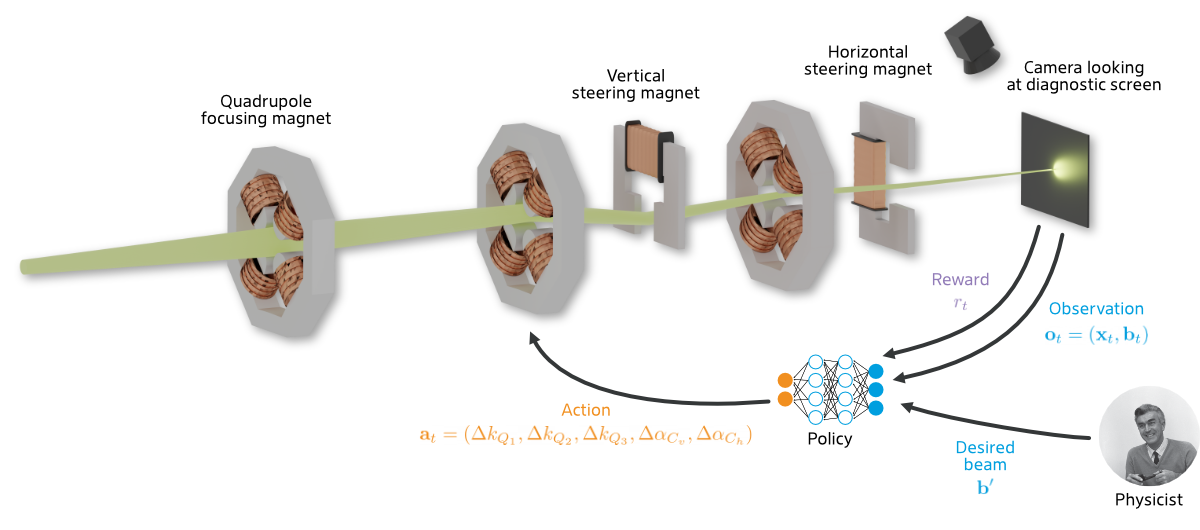
Learning-based Optimisation of Particle Accelerators Under Partial Observability Without Real-World Training
J. Kaiser, O. Stein, A. Eichler Deutsches Elektronen-Synchrotron DESY 39th International Conference on Machine Learning Abstract In recent work, it has been shown that reinforcement learning (RL) is capable of solving a variety of problems at sometimes super-human performance levels. But despite continued advances in the field, applying RL to complex real-world control and optimisation problems has proven difficult. In this contribution, we demonstrate how to successfully apply RL to the optimisation of a highly complex real-world machine – specifically a linear particle accelerator – in an only partially observable setting and without requiring training on the real machine....