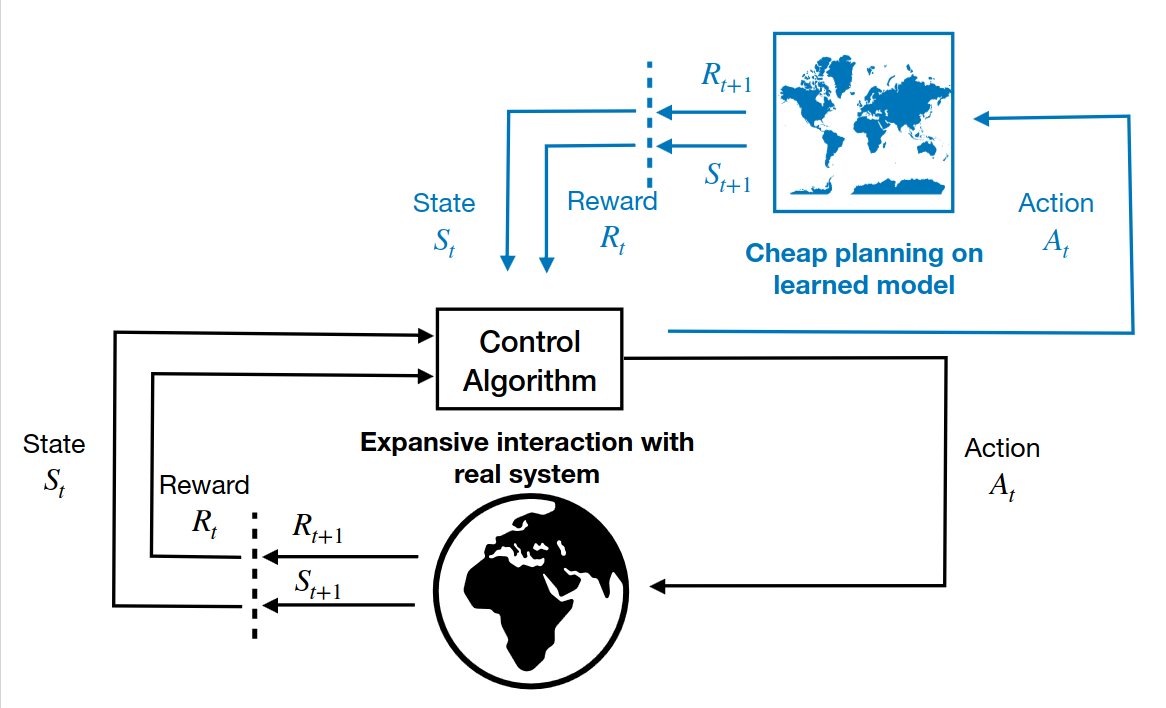
Ultra fast reinforcement learning demonstrated at CERN AWAKE
** Simon Hirlaender, Lukas Lamminger, Giovanni Zevi Della Porta, Verena Kain** Abstract Reinforcement learning (RL) is a promising direction in machine learning for the control and optimisation of particle accelerators since it learns directly from experience without needing a model a-priori. However, RL generally suffers from low sample efficiency and thus training from scracth on the machine is often not an option. RL agents are usually trained or pre-tuned on simulators and then transferred to the real environment....