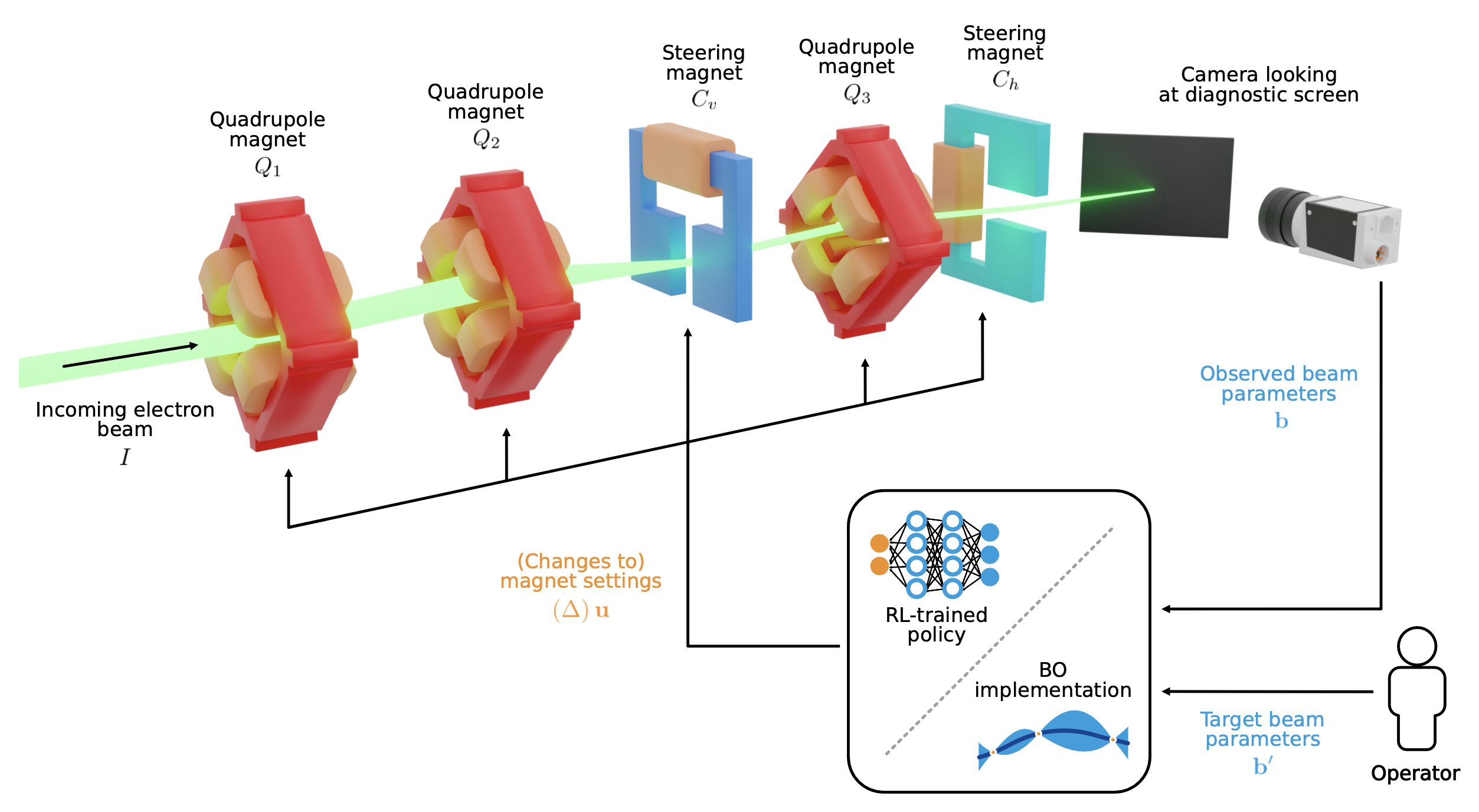
Learning to Do or Learning While Doing: Reinforcement Learning and Bayesian Optimisation for Online Continuous Tuning
J. Kaiser1, C. Xu2, A. Eichler1, A. Santamaria Garcia2, O. Stein1, E. Bründermann2, W. Kuropka1, H. Dinter1, F. Mayet1, T. Vinatier1, F. Burkart1, H. Schlarb1 1Deutsches Elektronen-Synchrotron DESY, 2 Karlsruhe Institute of Technology KIT arXiv Abstract Online tuning of real-world plants is a complex optimisation problem that continues to require manual intervention by experienced human operators. Autonomous tuning is a rapidly expanding field of research, where learning-based methods, such as Reinforcement Learning-trained Optimisation (RLO) and Bayesian optimisation (BO), hold great promise for achieving outstanding plant performance and reducing tuning times....